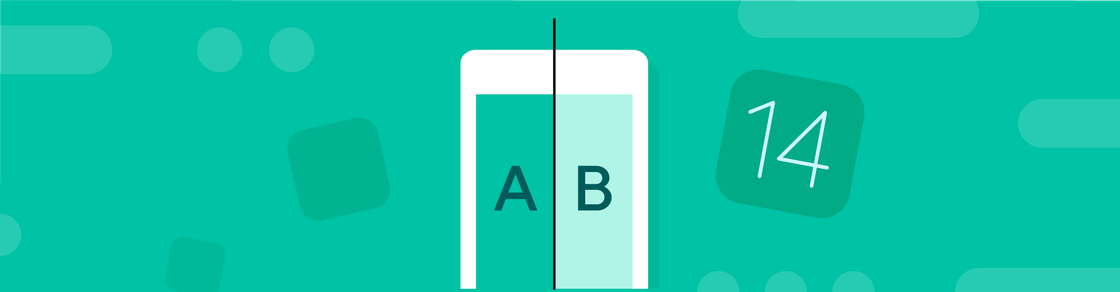
User trust and the ATT opt-in: A/B testing best practices for iOS 14.5+

Tiahn Wetzler, Director, Content & Insights, Adjust, Sep 02, 2022.
Since the announcement of Apple’s iOS 14 in 2020 through to the rollout of iOS 14.5 in April 2021 and the recent announcement of iOS 16, Adjust has been busy researching strategies and building solutions for success. From best practices for getting the opt-in to helping clients implement robust SKAdNetwork (SKAN) and conversion value strategies, our industry has pivoted to embrace a new privacy-centric model of data analysis and attribution.
In this series, we look at the opt-in and how you can optimize to drive consent rates up. High App Tracking Transparency (ATT) opt-in rates don’t just mean more consented data for granular analysis. The consented information you’re able to extract from devices that have provided access to the IDFA make up essential building blocks for your broad SKAN strategy and for how you work with conversion values and predictive analytics.
Today, we’re taking a look at A/B testing in relation to the opt-in. For a quick refresher, we also recommend taking a look at the first two parts of this opt-in series: Design do’s and don’ts and Securing user consent.
User attitudes towards data privacy
Encouragingly, research suggests that many consumers are more comfortable with personalized advertising than initially thought. One study performed by Oxford Economics suggests that around 70% of consumers are open to opting-in for a tailored ad experience. The data shows that only a small minority are against a more personalized, targeted experience:
- Just 17% feel uncomfortable with personalized offers
- Only 15% feel uncomfortable with personalized products and services
Trust is a huge factor that plays into how comfortable users are with opting-in. Another study, conducted by Salesforce, asked over 8,000 consumers what trust means. 75% responded with “privacy”, and 70% with “transparency” - showing the extent to which trust is interlinked with privacy and the clarity with which privacy policies are communicated.
The key takeaway here for mobile marketers is how essential it is to communicate the value of opting-in. With high proportions of consumers comfortable with personalized advertising, this is the key point that should be emphasized. Opting-in means receiving a personalized user experience with less frequent, more relevant ads. With opt-in rates creeping up according to Adjust data, it seems that the industry’s messaging around the value-add of opting-in is improving and that consumer awareness regarding what the ATT prompt actually is is also on the rise.
Best practices for privacy notices
Privacy notices are typically used by organizations to explain how they process personal data and how it applies to different data protection policies, such as the GDPR.
To better understand how privacy notices are presented on mobile, Adjust ran an audit of common practices. We saw three trends in how information is usually presented:
- Some apps allow users full data control, with the most granular opt-in options
- Some apps limit control by not listing all partners they work with
- A third group of apps use an “all in” or “all-out” approach, where users are allowed to either accept sharing data with all enlisted parties or none of them.
We will always recommend clients go with the first approach: be as transparent as possible, and give users full control over their data. To support this, you can explain the reason behind data collection through compelling copy and illustrations as well as outlining the positive benefits of opting-in.
Grouping
When iOS 14 was first announced, many brands were wondering whether it would be possible to serve the ATT prompt request grouped alongside other privacy notices. While you can customize a pre-permission prompt, the ATT prompt itself is set, only the second string is customizable and no additional notices can be included. It’s also worth noting that under GDPR, users cannot be directed into a given reply by pre-selecting opt-in checkboxes or making the opt-in CTA a primary button.
One study has shown that proper framing of the consent message positively impacts opt-in rates: if there are two options to give consent and the message is framed positively, users are more likely to opt-in - such as in the example below. This kind of positive framing is an important point to keep in mind around privacy notices in general. You want to emphasize the benefits.
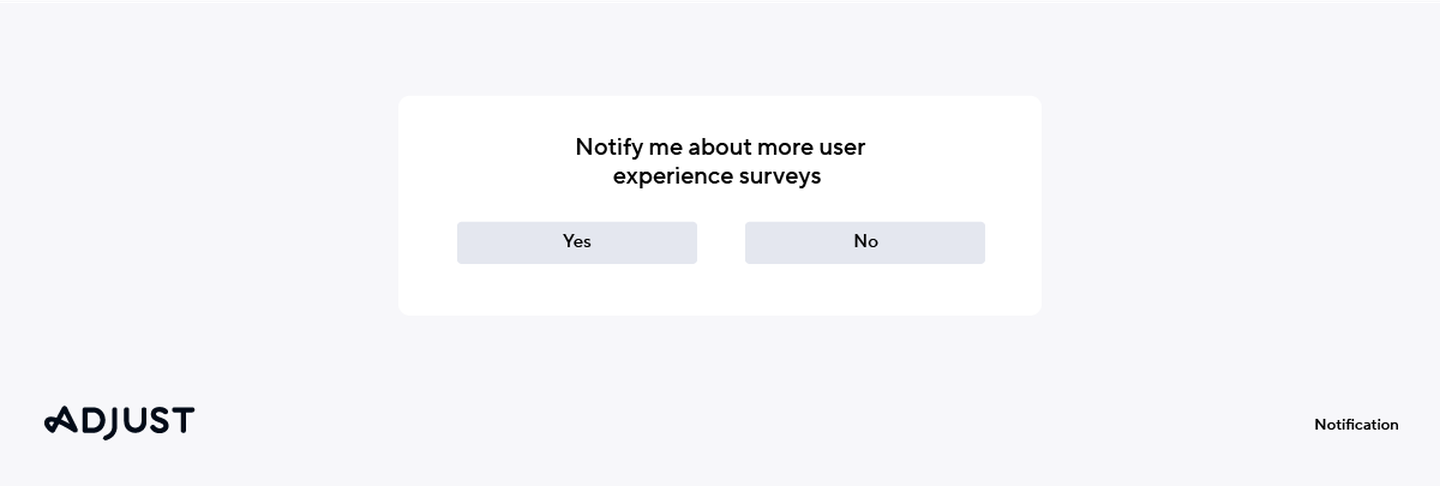
Evaluating your ideas: A/B testing
A/B testing is a great way to evaluate your solution by comparing two opt-in strategies and assessing their success.
To start, we recommend you A/B test both bundling your opt-in message with GDPR privacy notice at pre-permission levels, presenting it as a standalone message. If a user accepts your opt-in message, don’t forget to then also simulate Apple’s ATT pop-up.
Below, we’ve outlined test rounds with different aspects that can help you define a research plan.

Second round of testing
You can then build on these results by introducing further variables. For example, if a bundled privacy notice that includes the Apple request is more successful, you can explore the effects of different copy or design on opt-in rates. Alternatively, if you find that displaying a standalone request (e.g. a pre-permission prompt or the Apple pop-up) was more successful, you can assess the timing of when it’s served.
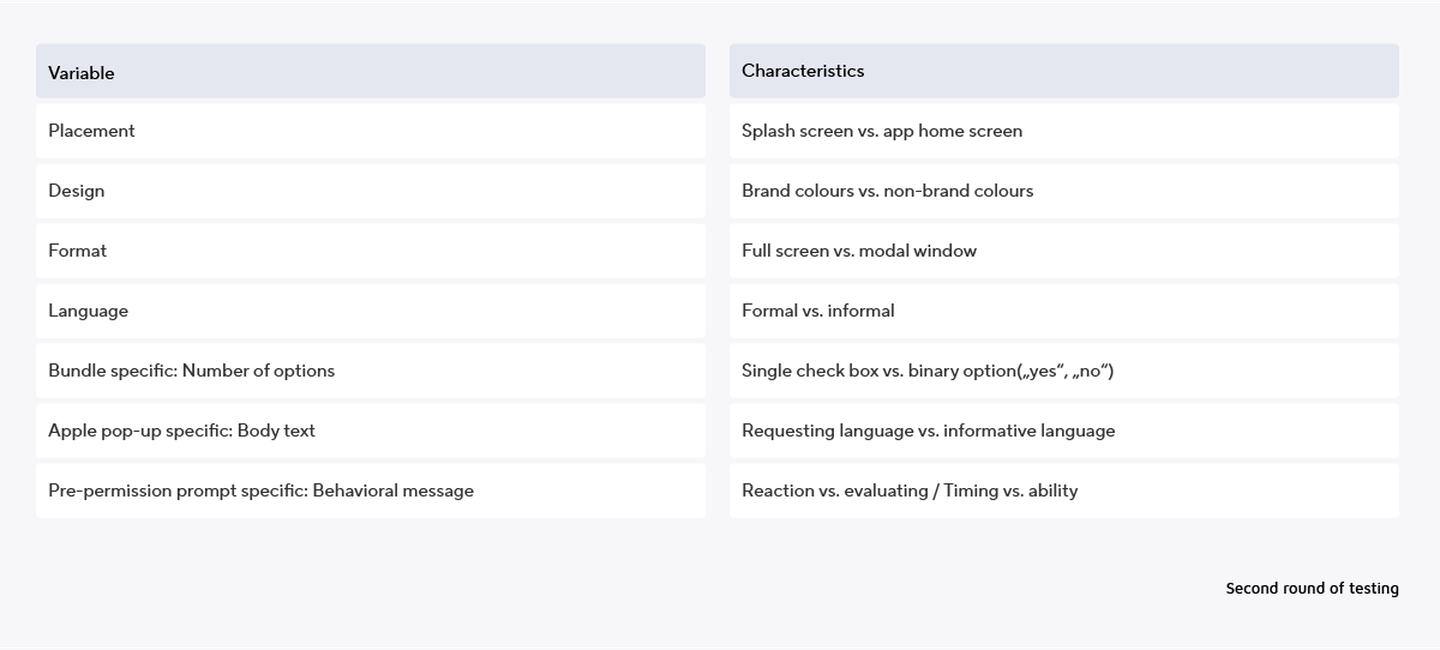
If you have a large user base and enough resources, you can also consider evaluating the effects of more than one variable on opt-in rates using log linear analysis. We also recommend evaluating the frequency of displaying your opt-in approach again for users who didn’t initially opt-in.
You can explore whether there are statistically significant effects for different user segments. You might find that the opt-in rates for new users are higher than for existing users, or that users from one region opt-out more than users from another. With this knowledge, you’re one step closer to dynamically adapting your strategy to further improve opt-in rates.
After any A/B testing, you should calculate a confidence interval to interpret the data. This helps determine what range the true opt-in rate would fall within if the test was conducted with every one of your app users.
Predict the opt-in: Predictive modeling
Predictive modeling uses statistical techniques to predict certain user behaviors. There are two types that can be helpful for analyzing your A/B tests:
- Regression analysis investigates the relationship between variables. It can be used to predict the value of an outcome variable based on predictor variables.
- Decision tree analysis is used to predict a target variable's outcome based on the observations of input variables.
With these analysis methods, you can investigate which variables are most influential in predicting a user’s response. Using certain contextual information, this lets you predict which of the two categories (opt-in vs. opt-out) a user likely belongs to. Here are some examples of predictor variables that you could use as a training data set:

Both logistic regression and decision tree analysis are good methods for solving classification problems. Logistic regression is generally the better approach if you believe that your data set divides linearly into two parts, one part associated with the decision to opt-in and the other with the decision to opt-out. You should also use regression analysis if the values of your predictor variables are continuous.
But if you’re unsure about the data separation, a decision tree is a better fit. And if your dataset contains a lot of outliers, missing values, or is skewed, a decision tree is also often the better choice.
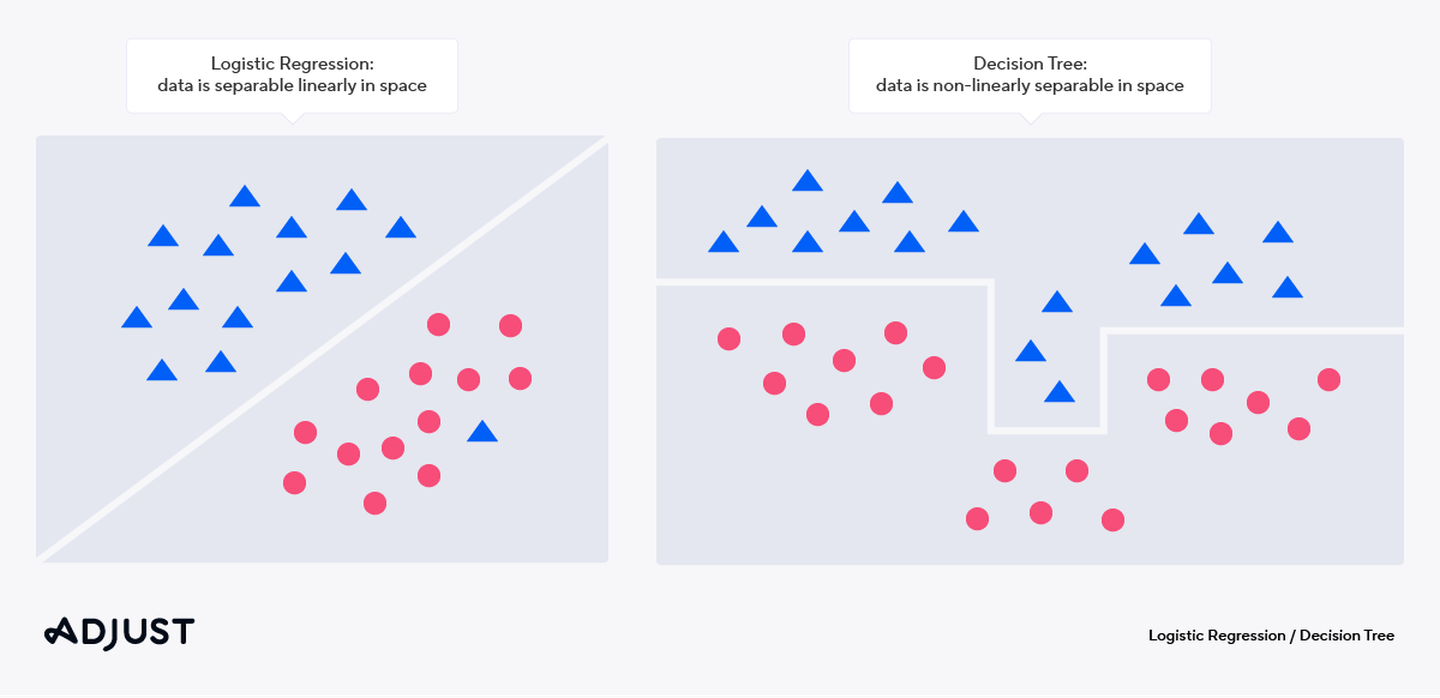
We recommend that you start by applying both methods and then decide which model gives the best result. As a next step, you can assess the individual contribution of the predictor variables to see which variables (e.g. install type, region, demographics etc.) have the biggest influence on the user decision.

Uncovering your consumer motivations: Speak to your users
A/B testing and regression analysis will show which factors are likely to increase the user opt-in rate - but these methods won’t tell you why the approach works and why specific variables are more important than others. Ultimately, this comes through speaking to your users, and conducting in-depth interviews that turn quantitative findings into defined decision paths. Insights from these interviews, both with users who have or are likely to opt-in and -out, will let you improve your dynamic opt-in strategy even further.
Summary
Data and user privacy is a transformational subject in the world of mobile and app marketing. At Adjust, we strongly believe this is something every company should have at the core of their approach to data and working with consumers. Ultimately, taking a clear and transparent approach will help build your app users’ trust, and make them more open to opt-in to sharing their IDFA.
At almost 18 months since the rollout of iOS 14.5, we’re seeing a slow and steady increase in overall opt-in rates. By testing and defining how to optimize your consent flow, you’ll consistently increase your chances of building understanding with your users and securing high opt-in rates. Get in touch with your Adjust contact person for more info!
Be the first to know. Subscribe for monthly app insights.